Human feedback for artificial intelligence: using RLHF to train your tech
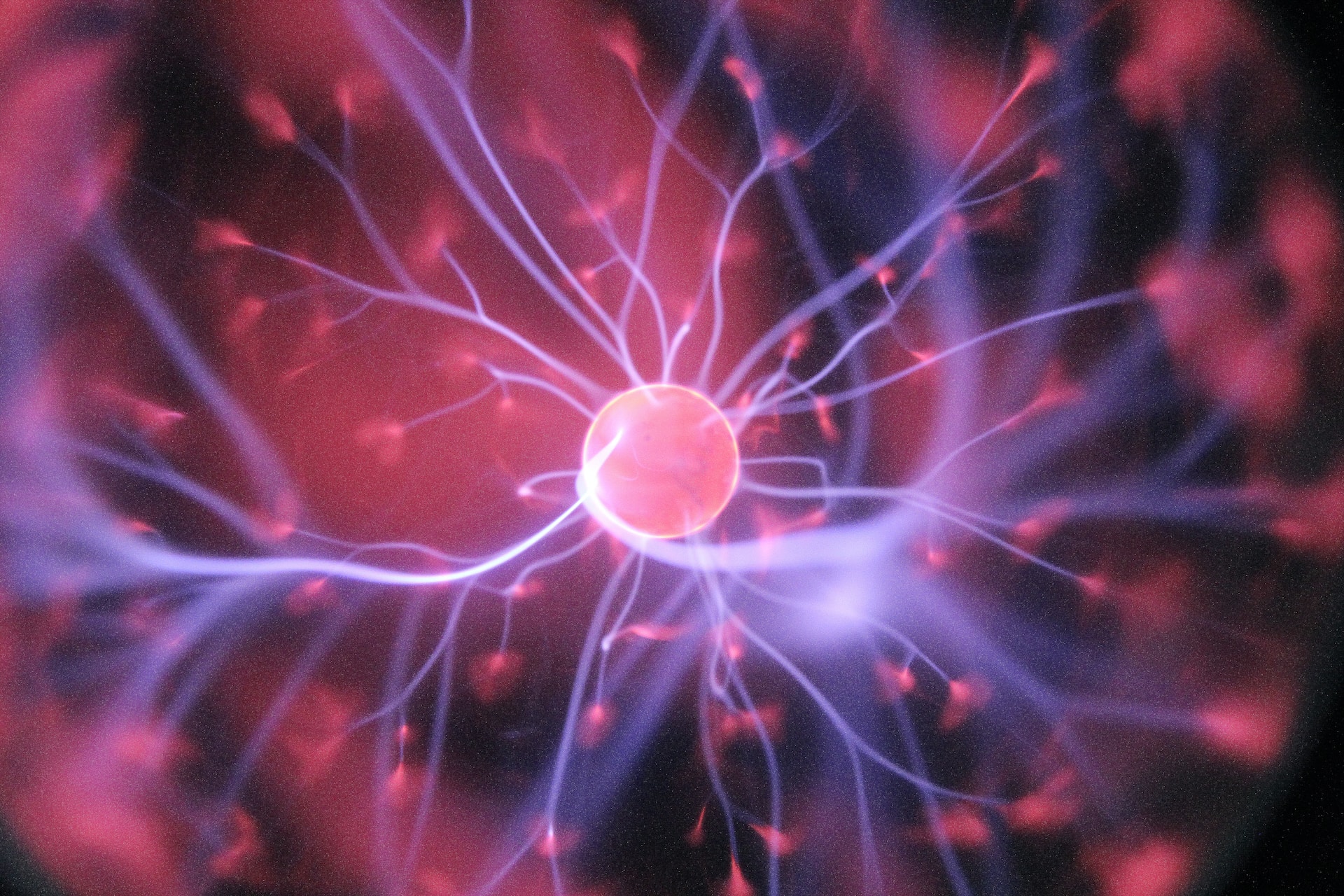
Spend more than five minutes online today, and you’ll find some mention of artificial intelligence (AI) – or even content created using it.
It’s no wonder it’s such a hot topic. AI has come a long way in recent years, evolving from a futuristic dream to a banded-around buzzword to a usable reality.
And it’s not just for the technically minded, either. Today, the average joe can rustle up a factually and grammatically correct, if slightly bland, article or blog post using a tool like ChatGPT. (This one was penned by real people. Honest.)
One of the most promising AI developments – and a potential way to brighten up that blandness – is reinforcement learning through human feedback (RLHF).
Introducing RLHF
The RLHF approach involves creating a machine-learning model and then continuing to educate it by asking for human feedback or input. This could mean getting people to score an AI chatbot’s response using various criteria, for example. How funny was the chatbot? How natural sounding? How informative?
In reinforcement learning (RL), AI agents are trained on a reward and punishment mechanism. So, they’re rewarded for correct moves and punished for wrong ones – and therefore incentivized to get responses right every time.
Then, in RLHF, human feedback is added, whereby real-life annotators compare various outputs from the AI agent and pick those they prefer: responses that are closer to the intended goal.
By combining the elements of traditional RL and plenty of input from the right people, AI can complete tasks with super-human performance.
The importance of human feedback
RLHF is crucial for the future of AI, as it allows machines to learn from human experiences and make better decisions in the real world.
Without human feedback, AI models can only learn from pre-existing data. This limits their ability to adapt to new situations or environments. With RLHF, however, machines can receive feedback in an instant – allowing them to improve their performance and refine their output with every request.
RLHF also aligns AI models’ objectives with our desired end behavior. Usually, we don’t want agents to imitate humans – we want them to give high-quality answers. This mismatch becomes evident when a model is trained to create low-quality text in a humanized voice, but it can happen in more subtle ways as well.
For example, a model trained to predict what a human would say might make up facts when it’s unsure or generate sentences reflecting harmful social bias. Both of these problems have been well-documented by OpenAI: the platform that powers ChatGPT.
OpenAI trained a model to summarize text using human feedback. Then they refined its output based on the thoughts and opinions of human annotators. They found RLHF the best way to overcome this discrepancy, beating internet-based training, supervised fine-tuning and scaling up model size.
Artificial intelligence with real benefits
One of the most significant benefits of RLHF is that it allows machines to learn from the diverse experiences and perspectives of human beings.
This is critical in fields like healthcare, where AI models must be able to make decisions that are both accurate and ethical. By learning from human feedback, these models can make choices that reflect the values and priorities of diverse populations.
Another area where RLHF has shown promise is in the development of autonomous vehicles. By training models on human feedback, they can make split-second decisions that might save lives. Think avoiding collisions or navigating through traffic, keeping passengers and other road users safe.
What’s more, self-driving cars can be taught to park by learning automatic parking policies, change lanes by learning some basic rules of the road, and overtake by learning how to do so while avoiding collisions and returning to a steady speed.
AI trained using RLHF can make useful contributions in the workplace, too. It can predict sales and stock prices across trading and finance, bid intelligently in real time across marketing and advertising, and even control light, heat, and electricity usage. In fact, Deepmind is currently used to cool Google’s data centers, which has driven a 40% reduction in energy spending.
Difficulties and drawbacks
But implementing RLHF is not without its challenges. One of the biggest obstacles is finding ways to incentivize human feedback.
Unlike typical data-labeling tasks, RLHF demands in-depth and honest feedback. The people giving that feedback need to be engaged, invested, and ready to put the time and effort into their answers.
This is where platforms like Prolific can make a difference.
How Prolific can help
Prolific connects you with a diverse pool of participants who give high-quality feedback on AI models.
We’re uniquely positioned to support RLHF for many reasons, including our:
- Rich responses - Grab exceptionally detailed, accurate and honest free text.
- Automated workflows - Perform high-volume tasks faster using our API.
- Simple scalability - Run research with thousands of trusted people at once, or a few favorites over and over.
- Plug-and-play platform - Access participants quickly and easily, with no faff or fuss.
- Instant integration - Bolt on your own tools or third-party apps – just add the link.
- Care and conscience - Rest easy knowing all our participants are vetted carefully, paid fairly, and treated ethically.
With Prolific, you can carry out everything from quick, iterative batch tasks in small groups to large-scale tasks over longer periods. As long as you have a URL, you can connect with our participants for all sorts of use cases, including NLP, computer vision, speech recognition, and more.
Plus, our sample pool includes individuals with expertise in specific fields, such as healthcare or engineering, who can provide targeted feedback on models in those areas. We even offer over 250 free screeners so you can find exactly who you’re looking for.
Find out more about how Prolific’s 130,000+ participants can help you with human-in-the-loop tasks today.